Abhinav Chouhan on how to build a data-informed culture
What can we learn from the journeys of smart people? What decisions shaped their life and career? That’s what we uncover in One Thousand Paths, a series of conversations with professionals from diverse fields.
“A young data team has to show value quickly… you need an early win to convince leadership about why your work is important. This means having the discernment to pick up the right use cases at the right time.”
Meet Abhinav Chouhan, an analytics and strategy leader with 15+ years of experience across industries (banking, retail, travel & hospitality) and geographies (India, US, Middle East, and Southeast Asia). His areas of interest include customer loyalty, customer experience, and marketing analytics.
We’re delighted to kick off our new series, One Thousand Paths, with Abhinav as our first guest. In this insightful chat, he talks about building data culture, the evolution of data analytics, the role of storytelling when presenting data, and a lot more.
Prefer to listen? Catch this episode on Spotify.
On his journey so far
Can you tell us a little bit about your background and how you found your way into analytics?
I started out doing my B.Tech in Production and Industrial Engineering at IIT Delhi. Interestingly though, my final year project involved working on a decision support system for optimizing procurement processes. That was my first brush with analytics.
After graduating, I worked for a consulting company with a ringside view of hedge funds that use tech platforms to understand their portfolios and the market—in effect, they were using analytics, though they weren’t calling it that. Next, I worked at Blackrock, where we focused on pricing and valuations. Again, what we were doing was analytics, though that wasn’t the vocabulary back then.
After a brief entrepreneurial stint, I realized that I needed to hone my business and leadership skills, and chose to pursue my MBA from ISB Hyderabad. Since my MBA, I have worked on what you might call core analytics roles, across different sectors.
You used to head the Analytics Center of Excellence at American Express. What is the mandate of such a CoE?
If you look back, there have always been cycles of centralization and decentralization in analytics. At Amex, the analytics CoE was an effort at centralization. We had a core team that performed the same kind of work across 27 international markets. There were region-specific nuances of course —for example, analyzing the customers in Sweden and Japan would give us very different insights. However, there were many synergies across geographies too, and leveraging those was the motivation for creating the Center of Excellence.
Would it be fair to say that you were also setting up a cross-geography data culture?
Yes. There were data culture discussions within the CoE itself, and more importantly, conversations with our stakeholders to educate them about analytics. What is it that we could bring to the table, why they should be interested in our insights, and so forth.
Have there been situations where you had to work with incomplete or insufficient data? How do you tackle those?
Definitely. In fact, you will never find an analytics head saying that he/she has enough data! In my current role, one of the things my team does is target the right set of people with the right marketing offer. Typically, we look at historical data to create these offers. But when entering new industry partnerships, we don’t have any historical data.
In such situations, we turn to market research to understand what kind of people might be interested in our offerings and create personas around them. We also look at adjacent information. So for example, if this is the first time that we’re entering a partnership with a shoe company, we’d scout around for the most adjacent industry - perhaps fast fashion, to get some data. Besides these, we also rely on surveys—and many times, talking to the business teams can give us clues around what to do next.
So yes, while a cold start is a problem, there are next-best alternatives. What is more important in these situations, is that as soon as we do something, we have to do a fast follow and learn quickly. This loopback has to be even faster when there’s limited data.
On building a data culture in startups
Any lessons for a startup founder who is trying to build a data culture within their organization?
For a strong data culture to exist, I'd say, two or three things are key. First, and this has been absolutely true with the organizations I’ve worked with, as well as younger organizations—there has to be sponsorship for data culture right at the top. Your leaders need to be convinced that the work of the data team is valuable, and this conviction will percolate down the rungs.
The second thing is that educating your stakeholders about analytics is not something that you do once and then forget about. You have to be persistent and consistent about propagating data literacy and training your stakeholders. It is a program that has to be managed consistently, with direct sponsorship from the top.
Lastly, data culture is essentially decision culture. What I mean is, data analysis is only important if it’s used for decision-making. Only then will a culture form around it. There could be individuals or teams that are doing fantastic work in silos, but if their work is not bonded to decisions, their relevance is under question.
Data culture is essentially decision culture. Data analysis is only important if it’s used for decision-making. Individuals or teams could be doing fantastic work in silos, but if their work is not bonded to decisions, their relevance is under question.
That’s a great way to put it! How can startup founders without a tech/data background create a data-driven culture?
Should you call it a data-driven culture? I prefer the term data-informed culture. The difference with a data-informed approach is that, as a leader, you would look at all the insights that your data team is providing, but you can choose to juxtapose your experience on top of that to arrive at decisions.
As a business leader, you do not need to understand the ins and outs of analytics. What is important is that you should know exactly what you're doing, and you need to know that your analytics team is rowing in the same direction. For this to happen, the analytics team has to have a seat at the table. This is why, about 15 years ago, there was the rise of a role called the Chief Information Officer, which is now evolving to a CDAO (Chief Data & Analytics Officer).
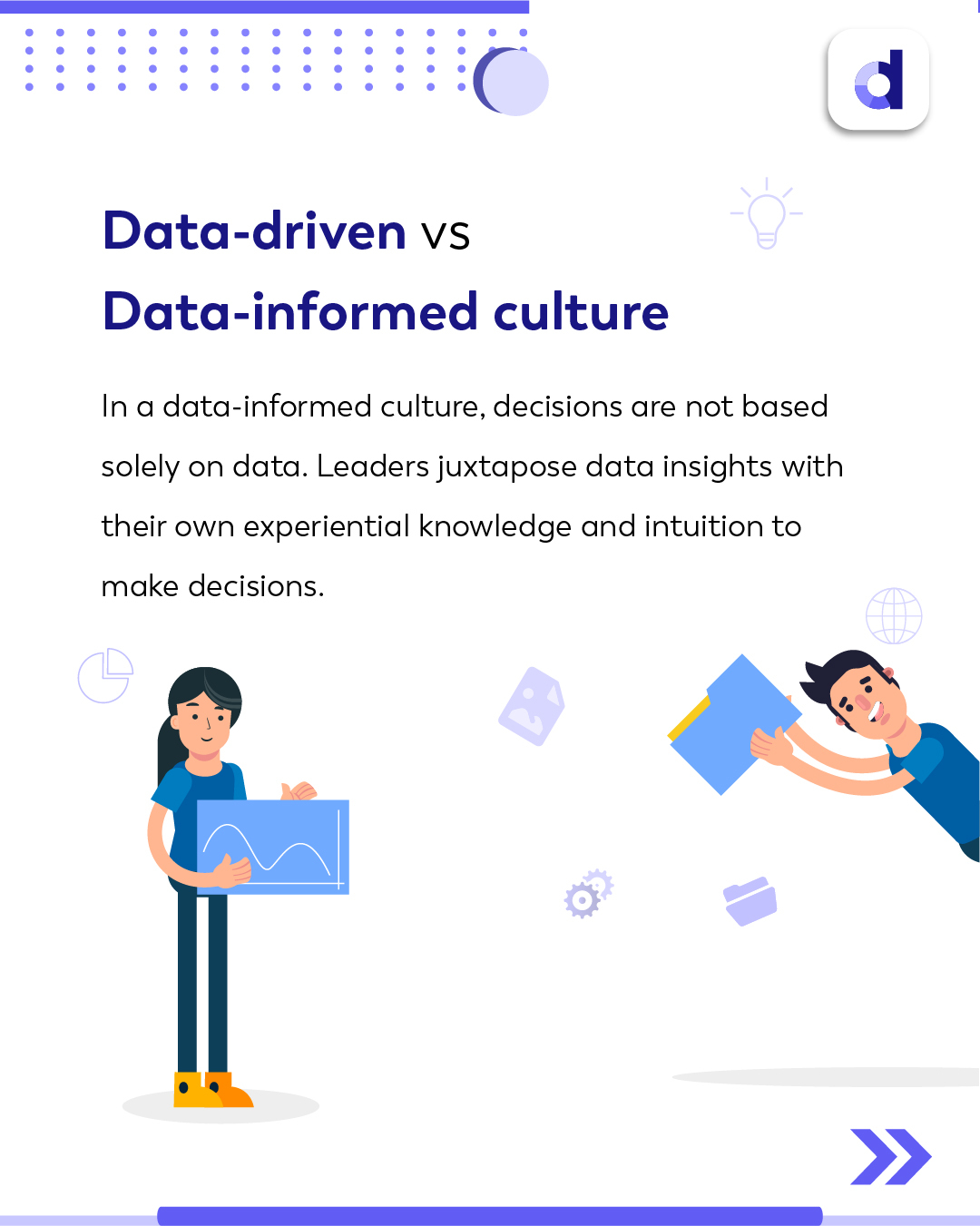
Across your various stints, what are some things that contributed to the success of the data-informed culture? Did you find any common factors?
The first thing, by far, and we spoke about this earlier—there has to be an acknowledgement from leadership that analytics is valuable. You’d think that creating the best model is the most important thing. But I believe the acknowledgement that yes, this particular team is valuable and we should listen to them—that is the most crucial thing for a data team to thrive.
Second, and this is reciprocal to the first point: a young data team has to show value quickly. This needn’t be something huge, like a million dollars saved, but you need an early win to convince leadership about why your work is important. This also means having the discernment to pick up the right use cases at the right time, something in keeping with the size and capabilities of the data team.
A young data team has to show value quickly… you need an early win to convince leadership about why your work is important. This means having the discernment to pick up the right use cases at the right time.
The third thing I’ve seen underpinning most successful data cultures, is just keeping it simple! People often think of analytics as the sort of field where the most complex thing should be the best thing, but that is rarely the case. The solutions that sell best are the ones that are simple and explainable to the stakeholders.
‘The Bull’, an artwork by Picasso, has 12 renditions of a bull, from the most detailed drawing to a deconstruction that is just 4-5 strokes. The takeaway from this work is abstraction: learning how to simplify complex subjects down to their basics. In analytics too, to get to simplicity, we have to go through this process.

On storytelling with data
Now that you’ve mentioned selling, I'm thinking about what role storytelling plays in data analytics. How do you see it?
Storytelling is everything! No matter how many insightful, beautiful charts you have - it all just falls flat without a story to weave it together.
Any analysis is only as important as how well you've understood it. We have to understand what problem the stakeholder is trying to solve, and present our findings such that the actionables are crystal-clear. This is something that has increasingly been the focus of the industry as well.
Ten years ago, the focus was on, how do we create this beautiful chart? But the past 4-5 years have been about how we present insights. As for becoming good at storytelling in analytics, there is no course that can teach you that overnight. I think the key is to just constantly be in touch with the stakeholders and really try to understand what they’re trying to solve for. Once you know that, you’ll know how to present the data.
Any analysis is only as important as how well you've understood it. We have to understand the problem the stakeholder is trying to solve, and present our findings such that the actionables are crystal-clear. Storytelling is everything.
On the evolution of analytics
What are the major inflection points you’ve seen in your career? What do you think Analytics is moving towards?
One important shift has been the democratization of analytics. The earliest practitioners were very technical. They would lock themselves up in a room for 32 hours and come out for a very beautiful, detailed chart. They had very valuable, very specialized skills.
Then, we moved towards the era of Tableau and Power BI, one step towards the democratization of insights. It was still the analytics team creating your dashboards, but the business user now had the capability of selecting a drop down, of slicing and dicing on their own.
I think the next step, which has already begun, with all the advancements in Gen AI, is that we’ll just ask what is happening and get the answer. In reality, it's never as simple as that. But I think that is the direction we’re headed in, and some players are already touting some of these things.
As a result, the analyst’s role changes too. Previously, we were focused on coding, creating dashboards etc. In the future, the analyst might be more focused with data quality. If we are feeding information to these giant models and expecting accurate answers, we better be sure of the inputs.
As we move more and more towards AI-driven models, analysts might need to focus more on data quality. The output is only as good as the input.
Another trend relates to analytics tools. Over the past 5-7 years, there’s been this Cambrian explosion of tools. Every other day, you wake up and see that another startup has got funding, with the result that we have, say, five different tools to do one specific thing. But I think that era is reaching its end. What I think we’re going to see next is consolidation in terms of tools.
On hiring data teams
For younger, fast growing, companies, what would you say is the right time to start building a data team?
I might be a little biased, but I’d say, right from the start, as soon as you start selling! Data teams can bring in different kinds of value, throughout the lifecycle of the company.
At the outset, you do not need a whole host of people - data engineers, dashboard developers, data scientists and such. But, as soon as the company goes live, there is data that has started coming through, so you do need a team, small as it may be. At this point, what is immensely helpful is a couple of data generalists, who can figure out how to do different things, rather than a team of specialists.
What is your personal hiring philosophy? What do you look for in a hire?
To me, a great hire is somebody who has a can-do attitude towards finding solutions and a knack for picking up things quickly. There are so many tools these days, chances are, the person I’m interviewing might not be well-versed with the tools we’re using. So I just look for somebody who likes and enjoys the learning process.
My other preference is that I don't look for people who are super technical. I would rather have someone who's good with the tools but also understands the business.
On life & learning
Now a softer question, just to get to know you a little better. What shows, books, and podcasts are you currently enjoying?
I find the show ‘The Last of Us’ quite gripping, an interesting take in the doomsday genre. As far as books go, I decided early this year that one of my focus areas is to be more productive. Two books I’m enjoying are Atomic Habits and Building a Second Brain.
‘Building a second brain’ resonated a lot with me and I have started implementing the central idea. Basically, the book helps you form a regimen to retain and remember the things you consume. I go through YouTube and blogs like there’s no tomorrow, but very often I forget the important points after a few days. The author says that this is because our brains are like RAM memory, meant for heavy processing, but not for storing things. So there has to be a second brain to store everything you learn—in other words, a tangible way to organize your knowledge.
As for podcasts, I listen to them regularly during my drive to work. I follow many analytics podcasts and a few others too— Revisionist History by Malcolm Gladwell comes to mind.
Any parting advice for founders and decision makers, as regards analytics?
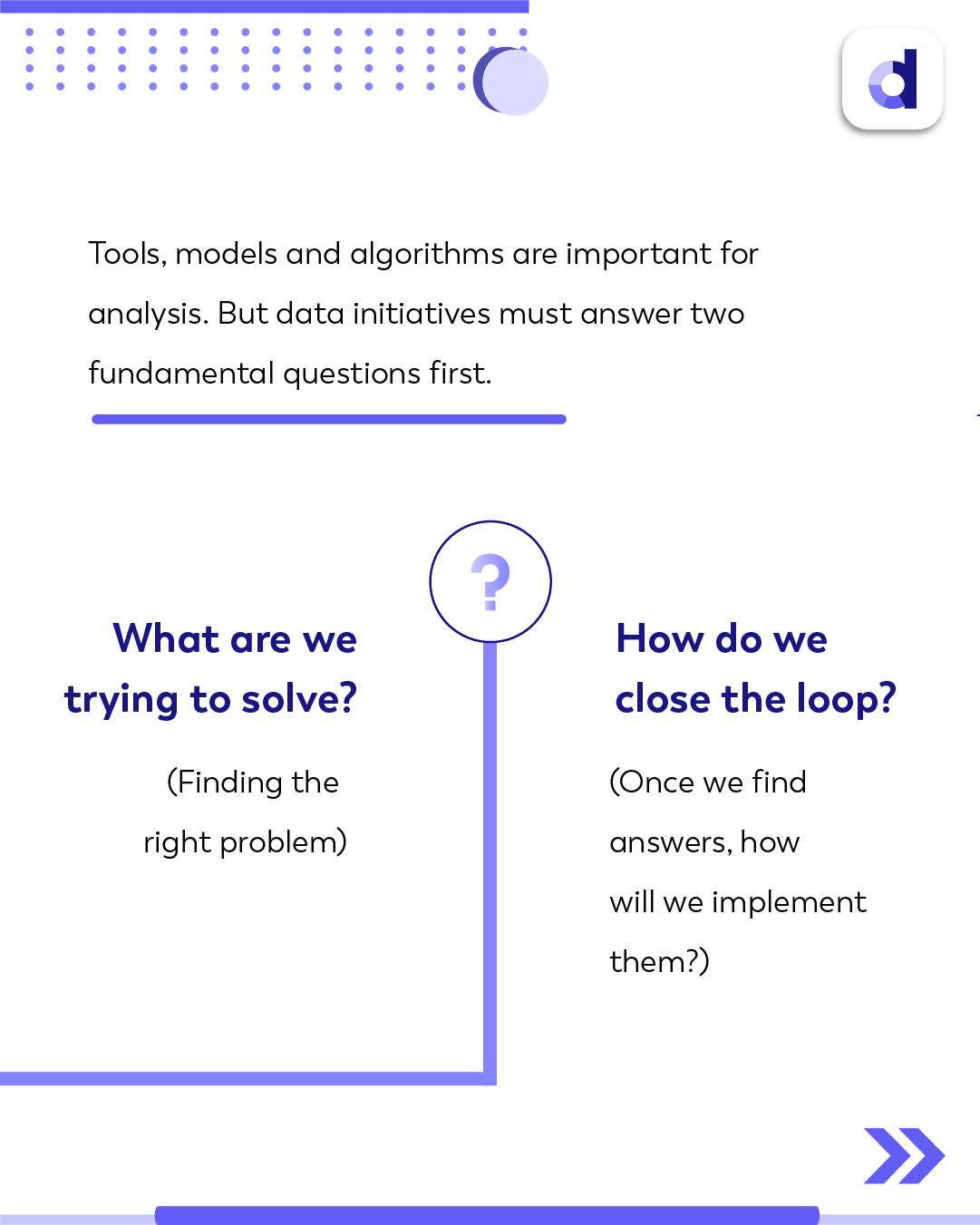
What I have learned over these years is that every data initiative should spend adequate time answering this—What are we trying to do? Are we asking the right questions?
Often, we find ourselves obsessing over the details, whether we should use tool A or tool B, etc. While tools, models, and algorithms are all important, the most incremental value comes from just understanding the ‘what’ of the problem and closing the loop—that is, once we get the answer, this is how we would implement it.
I think these are the two most important concerns, and we don’t focus on them enough. Most of the discussions, even online on blogs, revolve around Tableau versus Power BI, Python versus R, etc. Instead, we should engage in more discussions around the very fundamentals of decision-making. This is the thought I’d like to leave you with.
We hope you found many interesting takeaways from this discussion, as we did. Let us know your take in the comments.